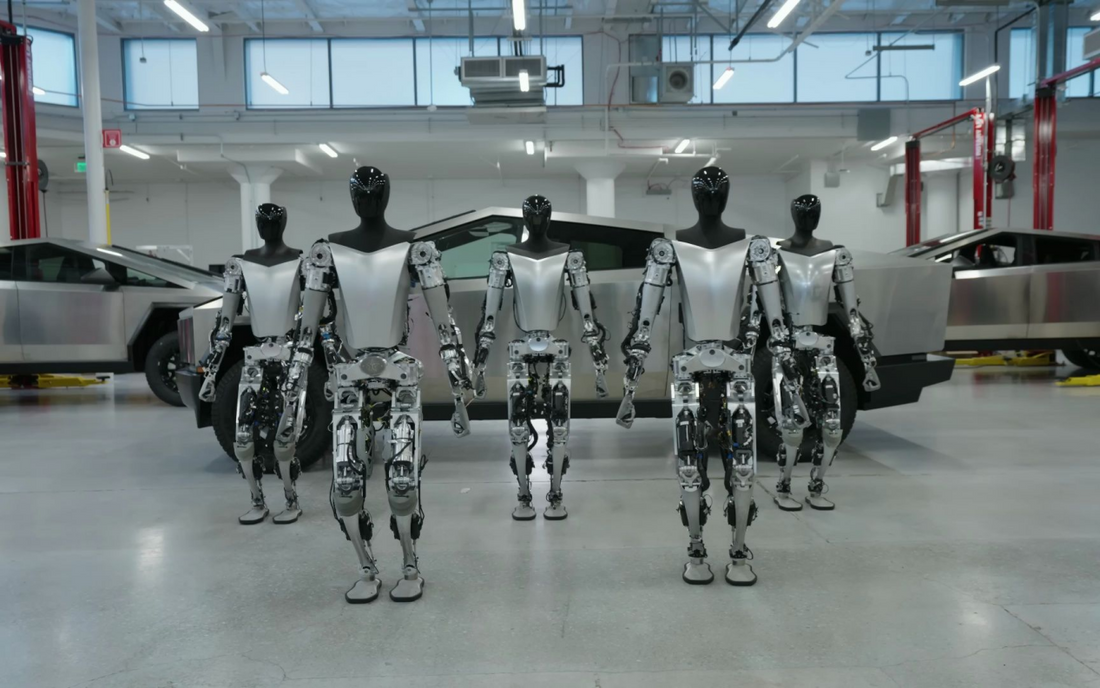
How to improve the perception, decision-making and interaction capabilities of humanoid robots
Share
In recent years, humanoid robots have gradually moved from science fiction concepts to reality. From Boston Dynamics' Atlas that shows difficult flips, to T's Optimus It is the one that moves parts in the factory, even the Japanese robot Pepper that speaks smoothly to humans, humanoid robots are overcoming technical bottlenecks and becoming a d Hot direction in the field of technology. However, for robots to truly integrate into human life, the central challenge is how to allow them to "perceive the environment", "think and t Omar decisions "and" interact naturally "like humans. Behind all this, artificial intelligence (AI) and machine learning (ML) technologies play a key role.
ⅠPerceived ability: from "sensory input" to "environmental understanding"
The perception ability of humanoid robots is a bridge to the physical world. Traditional robots rely on preset procedures to complete fixed tasks, while modern-shaped robots need to capture environmental information in real time through multi-modal sensors, and conduct dynamic analysis through machine learning models.

1. Visual perception: let the robot "understand" the world
Computer vision technology based on deep learning enables humanoid robots to recognize objects, faces, gestures and even emotions. For example, through a convolutional neural network (CNN), the robot can analyze the images captured by the camera in real time, distinguish between different objects (such as cups and books) and understand their spatial location.
Tesla vision system's Optimus combines multi-camera data and neural networks to enable 3D modeling of a complex factory environment to avoid obstacles and accurately capture parts.
2. Hearing and speech perception: understand instructions and emotion
Speech recognition technologies (such as RNN, Transformer models) allow robots to parse instructions, problems and even hidden intentions in human language. For example, SoftBank's Pepper robot can filter background noise in noisy environments through real-time voice processing to accurately identify user needs.
Further, the emotion recognition algorithm can judge the user's emotional state by analyzing the intonation, speed and keywords of the speech, so as to adjust the response strategy.
3. Tactile and force perception feedback: let the robot "perceive" the physical interaction
Touch sensors combined with reinforcement learning enable the robot to control the force of grasping. For example, the "Shadow Hand" manipulator developed by MIT is trained with tactile data to flexibly grab an egg or wrench without causing damage.
Force feedback technology also allows the robot to sense external thrust, such as adjusting its walking posture in a crowded environment to avoid collisions.
4. Multimodal data fusion
A single sensor data can cause misjudgment, but with multimodal machine learning (such as vision + speech + touch), the robot can understand the scene more fully. For example, when a user says, "Please give me the red box on the table," the robot needs to parse voice commands, locate the "table", identify the "red box", and plan the capture path.
Ⅱ Decision-making ability: from "mechanical execution" to "independent thinking"
Perception is the foundation, while decision-making is the "brain" of robots. Traditional robots rely on preset rules, but in dynamic environments (such as homes, public places), humanoid robots must make independent decisions through AI.
1. Reinforcement learning: Optimize your action strategy in trial and error
Reinforcement learning (RL) trains the robot to independently explore the optimal strategy, through the "reward mechanism". The Boston Dynamics' Atlas robot, for example, has learned to adjust its center of gravity and maintain its balance in rough terrain through millions of virtual simulation training sessions.
Tesla's Optimus uses RL to optimize the handling path, reducing energy consumption while improving task efficiency.
2. Scene understanding and path planning
Based on the algorithm of graph neural network (GNN), the robot can semantically segment complex scenes (such as distinguishing floors, walls, and movable objects), and plan safe paths in real time. For example, in a rescue scenario, the humanoid robot needs to determine the structural stability of the collapsed building and choose the best search and rescue route.
3. Imitation learning: from human "teacher"
By observing human movements (such as video or motion-capture data), robots can quickly learn complex skills. For example, OpenAI's Dactyl manipulator has mastered the ability to operate the Rubik's cube flexibly by mimicking human finger movements.
In a home scene, the robot can learn how to organize items or cook simple dishes by observing the owner's behavior.
4. Balance your long-term goals with your short-term movements
Humanoid robots need to combine both real-time tasks and long-term goals (such as "charging" and "avoid wear and tear"). Hierarchical reinforcement learning (HRL) and meta-learning (Meta-Learning) technologies are helping robots build multi-level decision frameworks to achieve more human thinking patterns.

Ⅲ Interaction ability: from the "cold machine" to the "emotional partner"
The ultimate goal of humanoid robots is to be a human partner, and natural interaction is the key to achieving this goal. AI technology is breaking down the "man-machine gap" and giving robots emotional resonance and social intelligence.
1. Natural language interaction: Contextual understanding in a dialogue
Large language models (such as GPT-4) give the robot contextual dialogue capabilities. The Ameca robot, for example, can combine conversation history to answer consecutive questions and even make humorous jokes.
Emotional language generation technology also allows the robot to adjust the tone to the scene, such as using gentle wording when comforting users.
2. Expression and body language: delivering emotional signals
Through facial expression recognition and generation technology, the robot can simulate smile, surprise and other expressions. For example, Engineered Arts's Ameca robot controls facial muscles through a tiny motor, achieve delicate expression changes.
Body language (such as nodding and gestures) is implemented through a motion planning algorithm to make the interaction more friendly.
3. Personalized interaction: Understand user preferences
Based on the clustering analysis of user behavior data, the robot can gradually learn the preferences of different family members. For example, provide educational content for children and remind the elderly of the time to take medicine.
Federated Learning (Federated Learning) technology also enables knowledge sharing across devices and improves robot adaptability while protecting privacy.
4. Social ethics and boundaries
AI needs to solve the problem of "terror valley effect" to avoid discomfort caused by excessive humanification. For example, the robot should keep a proper distance in the interaction to avoid the intrusion into the individual space.
Ethical algorithms can also help robots follow social norms, such as lowering the volume in public places and avoiding interfering with others.
Ⅳ Challenges and future prospects
While AI technology has greatly improved the capabilities of humanoid robots, the following challenges still need to be overcome:
Data dependence: Many models require massive amounts of annotated data, and the diversity of real scenarios is far greater than laboratory environments.Real-time requirements: complex decisions need to be completed in millisecond level, putting forward high requirements for computing power and algorithm efficiency.Lack of generalization: in unfamiliar scenarios, robots may fail as "never seen before."In the future, with the development of self-supervised learning, brain-computer interface, embodied intelligence (Embodied AI) and other technologies, humanoid robots may achieve the following breakthroughs:Less reliance on data: reduce the need for real data through physical simulation and transfer learning.Human-machine symbiosis: Robots can not only understand human instructions, but also proactively predict needs (such as preparing breakfast in advance).Emotional resonance: Through brain wave analysis or micro-expression recognition, the robot can deeply perceive human emotions and provide companionship.
From "perception" to "decision making" to "interaction", AI and machine learning are reconstructing the ability boundaries of humanoid robots. While there are still technological, ethical and commercial challenges ahead, it is foreseeable that future humanoid robots will not only be tools, but partners with understanding, creativity and empathy. This technological revolution may eventually blur the line between man and machine, but its core goal is always clear: to make technology serve the warmth of humanity.